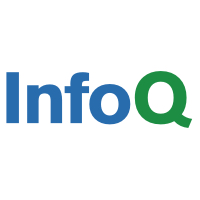
Rasa, an open up resource framework that supplies equipment mastering instruments to construct and deploy contextual AI assistants, recently held its developer summit in San Francisco. The speakers at the summit shared attention-grabbing company circumstance scientific studies on employing Rasa to establish AI assistants. “Our most important aim for the Rasa Developer Summit was to make community,” said Alex Weidauer, CEO & co-founder of Rasa. “We experienced speakers sharing the hottest analysis in conversational AI, lots of users of the group delivering authentic-entire world examples of contextual assistants in output, and absolutely everyone benefitting from the collaboration that will come in a group occasion,” Alex additional.
Alan Nichol, co-founder and CTO at Rasa, opened the summit by highlighting the need to have for conventional infrastructure to build level-3 contextual AI assistants. Akin to the 5 maturity levels of autonomous driving, the founders see 5 degrees of AI assistants.
Josh Converse, founder at Dynamic Offset, took to the stage to investigate the concept of a three component harmony that brings together Rasa, Kubernetes, and other disparate open up resource computer software (OSS) to establish an AI voice receptionist/assistant. He equated this harmony to how the Beatles, as a full, is bigger than the sum of its sections, the band customers. Josh discussed the architecture of the AI assistant and how the machine studying based dialogue management method, powered by Rasa, acts as the assistant’s mind. He showcased how Kubernetes can be leveraged to scale the AI assistant.
Edouard Malet, senior details scientist at N26, talked about the have to have to empower written content creators in buy to construct scalable AI assistants. Edouard pressured the fact that details experts may possibly lack the area know-how that is expected to style and design discussions carried out by an AI assistant. Following touching on the obligations of information researchers and content material creators, Edouard went on to go over the technological innovation stack and the pipeline––that encompasses Rasa, Jenkins, and Nomad––used at N26 to create scalable and sturdy AI assistants. He also discussed the troubles and alternatives to serve multilingual articles in a number of markets.
Nouha Dziri, investigation intern at Google AI, talked about the state-of-the-artwork entailment techniques that can be utilized to appraise coherence in open up finished dialogue devices. The obstacle, she explained, is to arrive up with an automated metric that can supply an precise evaluation of a dialogue procedure without the need of human enter. Nouha outlined how conversational logic can be modeled into four facets in terms of amount, high-quality, relevance, and manner. She closed by stating that scientists are even now on a quest to identify a dependable metric to examine open up finished dialogue programs.
Mady Mantha, Director of AI/ML at Sirius Computer system Methods, walked the audience by way of the evolution of Pure Language Processing (NLP). She talked about named-entity disambiguation (NED), the system of figuring out which object is referred to or meant by a named entity. A named entity is a actual earth object such as a man or woman, a location, or a amount. Acquire for instance this sentence “I am going to the lender.” “Financial institution” here can suggest both a river bank or a fiscal institution relying on the context in which it was stated. Mady showcased how Google’s BERT can be integrated with Rasa to deal with NED in a virtual vacation assistant. Supplied a person enter “e book me a ticket from London to Paris“, the BERT product was in a position to acknowledge and extract the resource (London) and spot (Paris) entities. The product outperformed other competing designs and was also in a position to take care of an input like “book me a ticket to Paris” and extract the location entity even even though the supply entity was missing from the user’s enter. Mady concluded her converse by highlighting the have to have for a continuous enhancement pipeline in get to build trusted AI assistants and what such a pipeline would entail.
InfoQ did a fast Q&A with Mady Mantha at the Summit.
InfoQ: 2018 observed a backlash on virtual assistants for failing to meet consumer anticipations. Do you see AI assistants building a comeback of kinds?
Mady: The backlash was reasonable. When individuals very first commenced interacting with virtual assistants, they generally as opposed it to human beings rule-based chatbots can’t contend with human beings at all, specifically when it comes to items like context switching, seamless job execution and in typical, the unpredictable character of human dialogue. A short while ago, we have observed a lot of progress in dialogue management methods and language models like Rasa’s Transformer Embedding Dialogue plan and Google’s BERT and ALBERT. These progress put together with tempered user expectations is promising. We can now begin to consider about how conversational AI can essentially add new income streams and decrease operational expenditures.
InfoQ: What are the crucial aspects to make and productize an AI assistant?
Mady: There are a large amount of components that go into earning a contextual assistant really effective. You may perhaps want to get started by defining the trouble house, for instance, is it a private vacation assistant, or it is an inner personnel-facing electronic assistant? Right after you build a sturdy NLP pipeline and discover integration points for process execution, establish a baseline to repeatedly appraise and increase your design based mostly on serious-user conversations and feed-back. The standards and best practices that use to setting up, deploying, and scaling software programs utilize to conversational AI for the most portion. It is significant to automate the entire workflow making use of a CI/CD pipeline, use a variation management process to take care of products, and execute holistic close-stop screening. Equipment that can assist with data tagging, dialogue enhancing and administration, version control, holistic tests, product re-evaluation and re-training based on actionable comments, essentially a continuous discovering cycle, can be particularly handy.
InfoQ: How can AI assistants increase current NLP purposes?
Mady: NLP is at the main of numerous text apps, like information retrieval, document classification, text summarization etcetera. For occasion, if a shopper is striving to return an buy, and lookups “how do I return an buy” in the textual content box, a backend technique can delegate the undertaking of answering this and related queries, together with aiding the shopper with buy returns to the contextual assistant. So, though an inverted index can be used to research for products, a contextual assistant can choose it to the subsequent amount by giving proactive purchaser treatment. In yet another example, you have an software that classifies legal documents. A contextual/legal assistant can classify and summarize documents, and in addition, present draft lawful briefs to paralegal groups for their critique.